Back to all members...
Yoav Gelberg
PhD, started 2024
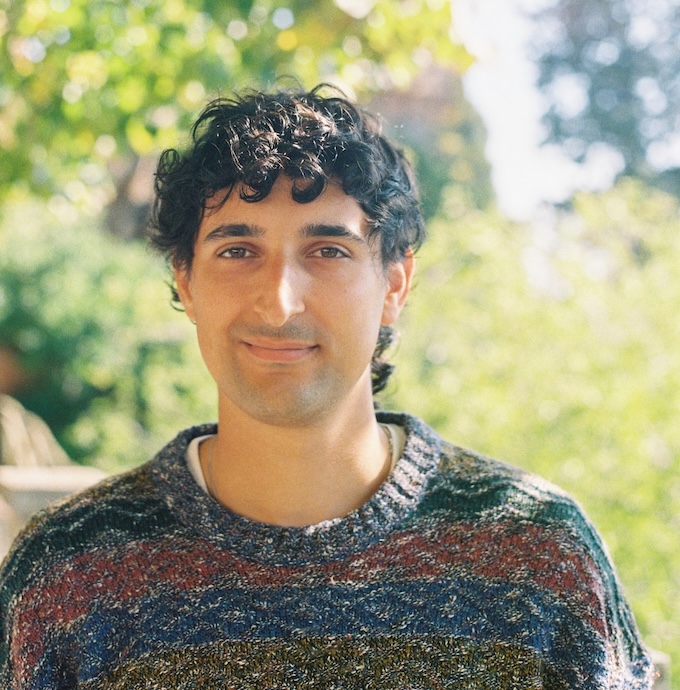
Yoav is a PhD student at the University of Oxford, co-advised by Yarin Gal, Michael Bronstein, and Haggai Maron. His research focuses on the symmetry and structure of neural network parameter spaces, with the goal of performing meta-learning tasks over model weights. He holds an undergraduate degree in mathematics from the Technion, where he was a Rothschild scholar. Before joining Oxford, Yoav conducted research on expander graphs at the Weizmann Institute, developed fair learning algorithms at Fairgen, and taught math and programming at the ARDC. His PhD is funded by the EPSRC through the AIMS CDT program.
Publications while at OATML • News items mentioning Yoav Gelberg • Reproducibility and Code • Blog Posts
Publications while at OATML:
Variational Inference Failures Under Model Symmetries: Permutation Invariant Posteriors for Bayesian Neural Networks
Weight space symmetries in neural network architectures, such as permutation symmetries in MLPs, give rise to Bayesian neural network (BNN) posteriors with many equivalent modes. This multimodality poses a challenge for variational inference (VI) techniques, which typically rely on approximating the posterior with a unimodal distribution. In this work, we investigate the impact of weight space permutation symmetries on VI. We demonstrate, both theoretically and empirically, that these symmetries lead to biases in the approximate posterior, which degrade predictive performance and posterior fit if not explicitly accounted for. To mitigate this behavior, we leverage the symmetric structure of the posterior and devise a symmetrization mechanism for constructing permutation invariant variational posteriors. We show that the symmetrized distribution has a strictly better fit to the true posterior, and that it can be trained using the original ELBO objective with a modified KL regulariza... [full abstract]
Yoav Gelberg, Tycho F.A. van der Ouderaa, Mark van der Wilk, Yarin Gal
ICML Workshop on Geometry-grounded Representation Learning and Generative Modeling (GRaM), 2024
[paper]
News items mentioning Yoav Gelberg:
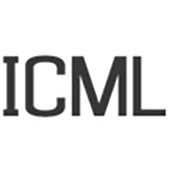
ICML 2024 GRaM workshop 'Best Paper' awarded
29 Jul 2024
A paper by Yoav Gelberg, Tycho F.A. van der Ouderaa, Mark van der Wilk and Yarin Gal received the top award in the Proceedings track of the ICML Workshop on Geometry-grounded Representation Learning and Generative Modeling (GRaM). You can read the paper on variational inference failures under model symmetries here.