Back to all members...
Gabriel Jones
Associate Member (PhD), started 2020
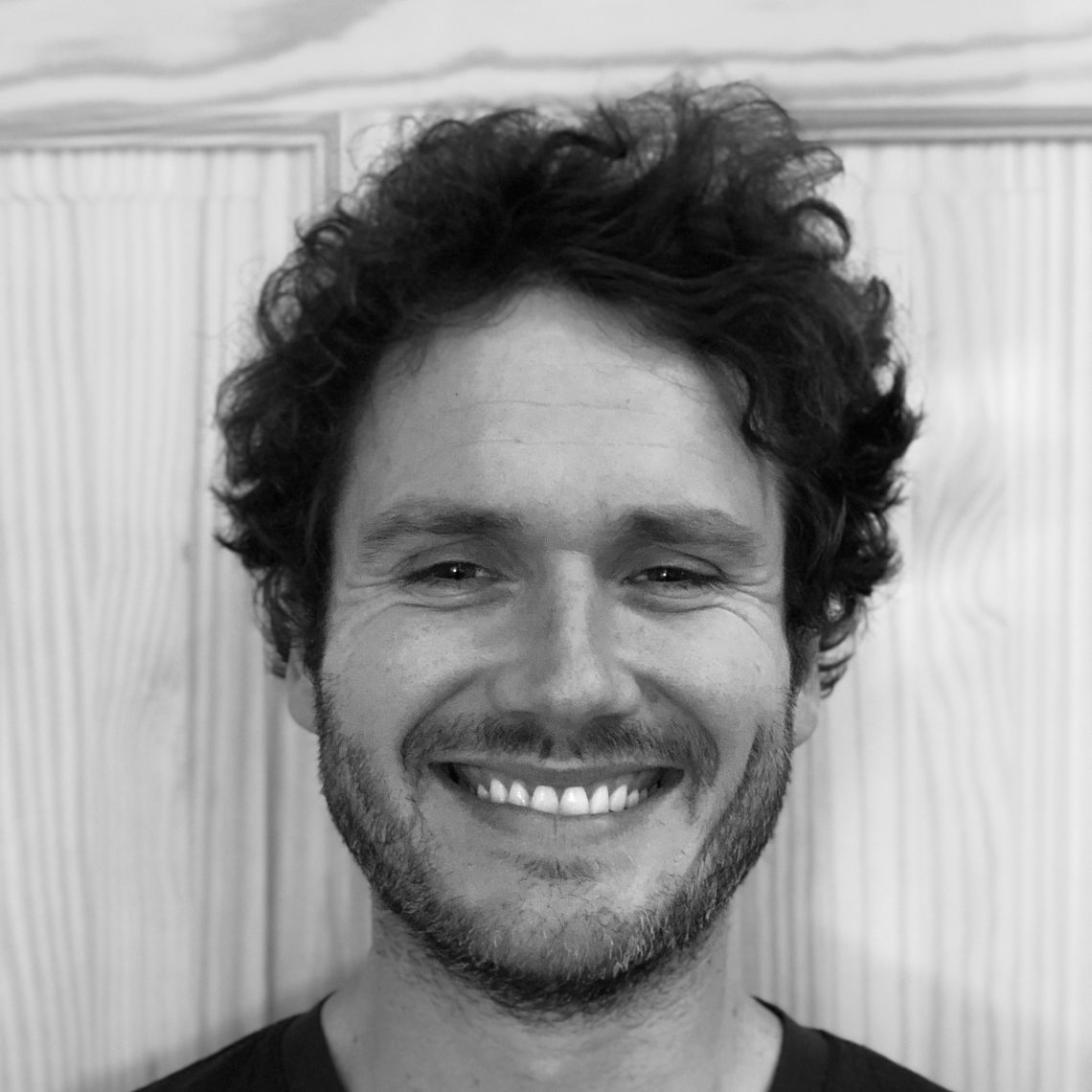
Gabriel is a doctoral candidate and Clarendon Scholar at the University of Oxford, supervised by Manu Vatish and Chris Redman, and co-supervised by Yarin Gal. He is interested in the theoretical foundations of machine learning and applying deep neural networks to clinical medicine. He obtained his Bachelors and Honours degrees from the University of Melbourne before completing a Doctor of Medicine (MD).
Publications while at OATML • News items mentioning Gabriel Jones • Reproducibility and Code • Blog Posts
Publications while at OATML:
Reducing Large Language Model Safety Risks in Women's Health using Semantic Entropy
Large language models (LLMs) hold substantial promise for clinical decision support. However, their widespread adoption in medicine, particularly in healthcare, is hindered by their propensity to generate false or misleading outputs, known as hallucinations. In high-stakes domains such as women's health (obstetrics & gynaecology), where errors in clinical reasoning can have profound consequences for maternal and neonatal outcomes, ensuring the reliability of AI-generated responses is critical. Traditional methods for quantifying uncertainty, such as perplexity, fail to capture meaning-level inconsistencies that lead to misinformation. Here, we evaluate semantic entropy (SE), a novel uncertainty metric that assesses meaning-level variation, to detect hallucinations in AI-generated medical content. Using a clinically validated dataset derived from UK RCOG MRCOG examinations, we compared SE with perplexity in identifying uncertain responses. SE demonstrated superior performance, achie... [full abstract]
Jahan C. Penny-Dimri, Magdalena Bachmann, William R. Cooke, Sam Mathewlynn, Samual Dockree, John Tolladay, Jannik Kossen, Lin Li, Yarin Gal, Gabriel Jones
arXiv
[paper]