Back to all members...
Lin Li
Postdoc, started 2025
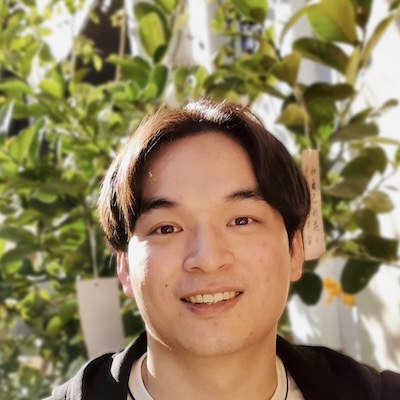
Lin is a postdoctoral researcher in the OATML Group at the University of Oxford, working under the supervision of Yarin Gal. His research focuses on AI Safety, including topics such as hallucination, jailbreaking, safety alignment, and adversarial machine learning. He is also interested in LLM-based agents, particularly in areas like autonomous complex decision-making and the simulation of human behaviour and societal dynamics. Beyond foundational research, Lin explores the application of machine learning to real-world challenges in domains such as healthcare, robotics, and economics.
Before joining Oxford, Lin completed his Ph.D. in Machine Learning from King’s College London, where his research centred on enhancing the robustness of machine learning models. He also interned at Tencent’s Robotics X Lab, where he worked on enabling robots to learn throwing and catching skills from human demonstrations.
Publications while at OATML • News items mentioning Lin Li • Reproducibility and Code • Blog Posts
Publications while at OATML:
Reducing Large Language Model Safety Risks in Women's Health using Semantic Entropy
Large language models (LLMs) hold substantial promise for clinical decision support. However, their widespread adoption in medicine, particularly in healthcare, is hindered by their propensity to generate false or misleading outputs, known as hallucinations. In high-stakes domains such as women's health (obstetrics & gynaecology), where errors in clinical reasoning can have profound consequences for maternal and neonatal outcomes, ensuring the reliability of AI-generated responses is critical. Traditional methods for quantifying uncertainty, such as perplexity, fail to capture meaning-level inconsistencies that lead to misinformation. Here, we evaluate semantic entropy (SE), a novel uncertainty metric that assesses meaning-level variation, to detect hallucinations in AI-generated medical content. Using a clinically validated dataset derived from UK RCOG MRCOG examinations, we compared SE with perplexity in identifying uncertain responses. SE demonstrated superior performance, achie... [full abstract]
Jahan C. Penny-Dimri, Magdalena Bachmann, William R. Cooke, Sam Mathewlynn, Samual Dockree, John Tolladay, Jannik Kossen, Lin Li, Yarin Gal, Gabriel Jones
arXiv
[paper]