Back to all members...
Gunshi Gupta
PhD, started 2021
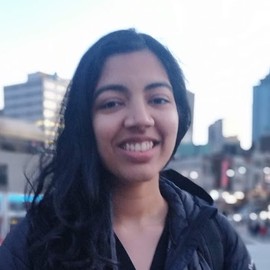
Gunshi Gupta is a DPhil student in the OATML group, supervised by Prof. Yarin Gal. She is interested in problems related to probabilistic and causally-correct learning from data, with a focus on robotics tasks. She grew up in New Delhi, India where she got her applied-math engineering degree from DTU. After dabbling in software engineering and robotics research, she went on to study machine learning at Mila supervised by Prof. Liam Paull. Her master’s research was on the topics of bayesian deep-learning, meta learning and continual learning. Before joining AIMS CDT, she worked at the autonomous driving startup Wayve that aims to solve the self-driving problem using end-to-end deep learning.
Publications while at OATML • News items mentioning Gunshi Gupta • Reproducibility and Code • Blog Posts
Publications while at OATML:
Pre-trained Text-to-Image Diffusion Models Are Versatile Representation Learners for Control
Embodied AI agents require a fine-grained understanding of the physical world mediated through visual and language inputs. Such capabilities are difficult to learn solely from task-specific data. This has led to the emergence of pre-trained vision-language models as a tool for transferring representations learned from internet-scale data to downstream tasks and new domains. However, commonly used contrastively trained representations such as in CLIP have been shown to fail at enabling embodied agents to gain a sufficiently fine-grained scene understanding -- a capability vital for control. To address this shortcoming, we consider representations from pre-trained text-to-image diffusion models, which are explicitly optimized to generate images from text prompts and as such, contain text-conditioned representations that reflect highly fine-grained visuo-spatial information. Using pre-trained text-to-image diffusion models, we construct Stable Control Representations which allow learn... [full abstract]
Gunshi Gupta, Karmesh Yadav, Yarin Gal, Dhruv Batra, Zsolt Kira, Cong Lu, Tim G. J. Rudner
ICLR Workshop on Generative Models for Decision Making, 2024
[paper]
Can Active Sampling Reduce Causal Confusion in Offline Reinforcement Learning?
Causal confusion is a phenomenon where an agent learns a policy that reflects imperfect spurious correlations in the data. Such a policy may falsely appear to be optimal during training if most of the training data contain such spurious correlations. This phenomenon is particularly pronounced in domains such as robotics, with potentially large gaps between the open- and closed-loop performance of an agent. In such settings, causally confused models may appear to perform well according to open-loop metrics during training but fail catastrophically when deployed in the real world. In this paper, we study causal confusion in offline reinforcement learning. We investigate whether selectively sampling appropriate points from a dataset of demonstrations may enable offline reinforcement learning agents to disambiguate the underlying causal mechanisms of the environment, alleviate causal confusion in offline reinforcement learning, and produce a safer model for deployment. To answer this q... [full abstract]
Gunshi Gupta, Tim G. J. Rudner, Rowan McAllister, Adrien Gaidon, Yarin Gal
CLeaR, 2023
NeurIPS Workshop on Causal Machine Learning for Real-World Impact, 2022
[OpenReview] [BibTex]
News items mentioning Gunshi Gupta:
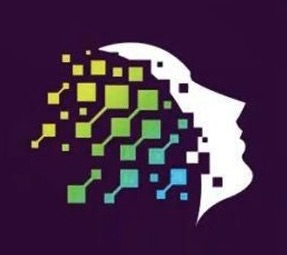
Gunshi Gupta presents work at ICLR 2024 GenAI4DM workshop
11 May 2024
OATML student Gunshi Gupta was invited to present recent work along with collaborators from Georgia Tech and NYU (including group member Tim Rudner) at the Generative AI for Decision Making workshop (GenAI4DM) at ICLR 2024. The talk was on the paper ‘Pretrained Text-to-Image Diffusion Models Are Versatile Representation Learners for Control’. Read the paper here.
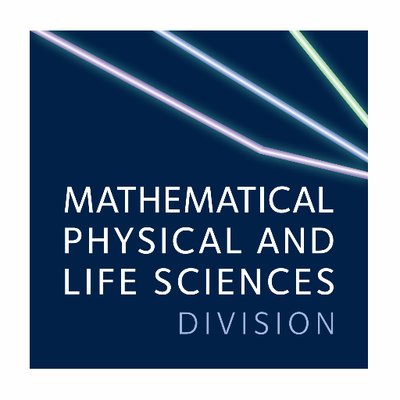
Gunshi Gupta is announced as Equality, Diversity and Inclusion Fellow
22 Sep 2022
OATML PhD Student Gunshi Gupta has been announced as one of the new Equality, Diversity and Inclusion fellows for the Mathematical, Physical & Life Sciences Division. Congratualtions to her, and all of the Fellows, who will help promote an inclusive and supportive culture for all.
Blog Posts
OATML Conference papers at NeurIPS 2022
OATML group members and collaborators are proud to present 8 papers at NeurIPS 2022 main conference, and 11 workshop papers. …
Full post...Yarin Gal, Freddie Kalaitzis, Shreshth Malik, Lorenz Kuhn, Gunshi Gupta, Jannik Kossen, Pascal Notin, Andrew Jesson, Panagiotis Tigas, Tim G. J. Rudner, Sebastian Farquhar, Ilia Shumailov, 25 Nov 2022