Back to all members...
Joost van Amersfoort
PhD (2018—2023)
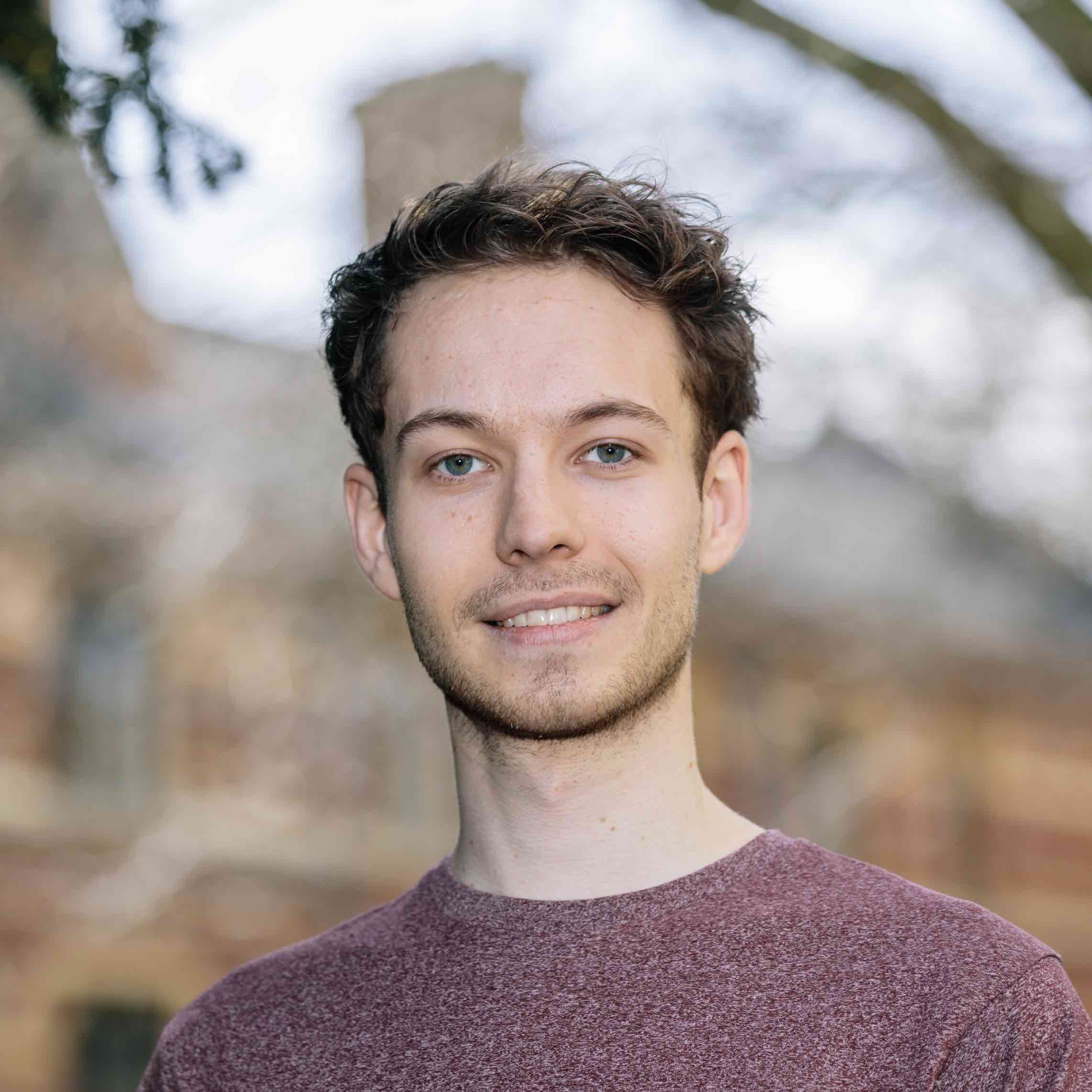
Joost was a DPhil student in the OATML group in the Department of Computer Science at the University of Oxford, supervised by Yarin Gal and Yee Whye Teh. He is interested in uncertainty estimation, representation learning, variational inference, and its applications to for example active learning. Previously, he spent two years as a Research Engineer at Twitter’s machine learning team Cortex as part of the team that came out of the Magic Pony acquisition. He obtained his MSc. at the University of Amsterdam, working with Max Welling and Diederik Kingma. He is an Oxford-Google DeepMind scholar.
Publications while at OATML • News items mentioning Joost van Amersfoort • Reproducibility and Code • Blog Posts
Publications while at OATML:
Mixtures of large-scale dynamic functional brain network modes
Accurate temporal modelling of functional brain networks is essential in the quest for understanding how such networks facilitate cognition. Researchers are beginning to adopt time-varying analyses for electrophysiological data that capture highly dynamic processes on the order of milliseconds. Typically, these approaches, such as clustering of functional connectivity profiles and Hidden Markov Modelling (HMM), assume mutual exclusivity of networks over time. Whilst a powerful constraint, this assumption may be compromising the ability of these approaches to describe the data effectively. Here, we propose a new generative model for functional connectivity as a time-varying linear mixture of spatially distributed statistical "modes". The temporal evolution of this mixture is governed by a recurrent neural network, which enables the model to generate data with a rich temporal structure. We use a Bayesian framework known as amortised variational inference to learn model parameters fro... [full abstract]
Chetan Gohil, Evan Roberts, Ryan Timms, Alex Skates, Cameron Higgins, Andrew Quinn, Usama Pervaiz, Joost van Amersfoort, Pascal Notin, Yarin Gal, Stanislaw Adaszewski, Mark Woolrich
NeuroImage
[paper]
Plex: Towards Reliability using Pretrained Large Model Extensions
A recent trend in artificial intelligence is the use of pretrained models for language and vision tasks, which have achieved extraordinary performance but also puzzling failures. Probing these models' abilities in diverse ways is therefore critical to the field. In this paper, we explore the reliability of models, where we define a reliable model as one that not only achieves strong predictive performance but also performs well consistently over many decision-making tasks involving uncertainty (e.g., selective prediction, open set recognition), robust generalization (e.g., accuracy and proper scoring rules such as log-likelihood on in- and out-of-distribution datasets), and adaptation (e.g., active learning, few-shot uncertainty). We devise 10 types of tasks over 40 datasets in order to evaluate different aspects of reliability on both vision and language domains. To improve reliability, we developed ViT-Plex and T5-Plex, pretrained large model extensions for vision and language mo... [full abstract]
Dustin Tran, Jeremiah Liu, Michael W. Dusenberry, Du Phan, Mark Collier, Jie Ren, Kehang Han, Zi Wang, Zelda Mariet, Huiyi Hu, Neil Band, Tim G. J. Rudner, Karan Singhal, Zachary Nado, Joost van Amersfoort, Andreas Kirsch, Rodolphe Jenatton, Nithum Thain, Honglin Yuan, Kelly Buchanan, Kevin Murphy, D. Sculley, Yarin Gal, Zoubin Ghahramani, Jasper Snoek, Balaji Lakshminarayan
Contributed Talk, ICML Pre-training Workshop, 2022
[OpenReview] [Code] [BibTex] [Google AI Blog Post]
Mixtures of large-scale dynamic functional brain network modes
Accurate temporal modelling of functional brain networks is essential in the quest for understanding how such networks facilitate cognition. Researchers are beginning to adopt time-varying analyses for electrophysiological data that capture highly dynamic processes on the order of milliseconds. Typically, these approaches, such as clustering of functional connectivity profiles and Hidden Markov Modelling (HMM), assume mutual exclusivity of networks over time. Whilst a powerful constraint, this assumption may be compromising the ability of these approaches to describe the data effectively. Here, we propose a new generative model for functional connectivity as a time-varying linear mixture of spatially distributed statistical “modes”. The temporal evolution of this mixture is governed by a recurrent neural network, which enables the model to generate data with a rich temporal structure. We use a Bayesian framework known as amortised variational inference to learn model parameters fro... [full abstract]
Chetan Gohil, Evan Roberts, Ryan Timms, Alex Skates, Cameron Higgins, Andrew Quinn, Usama Pervaiz, Joost van Amersfoort, Pascal Notin, Yarin Gal, Stanislaw Adaszewski, Mark Woolrich
NeuroImage Volume 263 [Paper] [BibTex]
On Feature Collapse and Deep Kernel Learning for Single Forward Pass Uncertainty
Inducing point Gaussian process approximations are often considered a gold standard in uncertainty estimation since they retain many of the properties of the exact GP and scale to large datasets. A major drawback is that they have difficulty scaling to high dimensional inputs. Deep Kernel Learning (DKL) promises a solution: a deep feature extractor transforms the inputs over which an inducing point Gaussian process is defined. However, DKL has been shown to provide unreliable uncertainty estimates in practice. We study why, and show that with no constraints, the DKL objective pushes "far-away" data points to be mapped to the same features as those of training-set points. With this insight we propose to constrain DKL's feature extractor to approximately preserve distances through a bi-Lipschitz constraint, resulting in a feature space favorable to DKL. We obtain a model, DUE, which demonstrates uncertainty quality outperforming previous DKL and other single forward pass uncertainty ... [full abstract]
Joost van Amersfoort, Lewis Smith, Andrew Jesson, Oscar Key, Yarin Gal
arXiv (2022)
[Paper]
Prospect Pruning: Finding Trainable Weights at Initialization using Meta-Gradients
Pruning neural networks at initialization would enable us to find sparse models that retain the accuracy of the original network while consuming fewer computational resources for training and inference. However, current methods are insufficient to enable this optimization and lead to a large degradation in model performance. In this paper, we identify a fundamental limitation in the formulation of current methods, namely that their saliency criteria look at a single step at the start of training without taking into account the trainability of the network. While pruning iteratively and gradually has been shown to improve pruning performance, explicit consideration of the training stage that will immediately follow pruning has so far been absent from the computation of the saliency criterion. To overcome the short-sightedness of existing methods, we propose Prospect Pruning (ProsPr), which uses meta-gradients through the first few steps of optimization to determine which weights to p... [full abstract]
Milad Alizadeh, Shyam A. Tailor, Luisa Zintgraf, Joost van Amersfoort, Sebastian Farquhar, Nicholas Donald Lane, Yarin Gal
ICLR, 2022 [arXiv] [OpenReview] [BibTex]
Causal-BALD: Deep Bayesian Active Learning of Outcomes to Infer Treatment-Effects
Estimating personalized treatment effects from high-dimensional observational data is essential in situations where experimental designs are infeasible, unethical or expensive. Existing approaches rely on fitting deep models on outcomes observed for treated and control populations, but when measuring the outcome for an individual is costly (e.g. biopsy) a sample efficient strategy for acquiring outcomes is required. Deep Bayesian active learning provides a framework for efficient data acquisition by selecting points with high uncertainty. However, naive application of existing methods selects training data that is biased toward regions where the treatment effect cannot be identified because there is non-overlapping support between the treated and control populations. To maximize sample efficiency for learning personalized treatment effects, we introduce new acquisition functions grounded in information theory that bias data acquisition towards regions where overlap is satisfied, by... [full abstract]
Andrew Jesson, Panagiotis Tigas, Joost van Amersfoort, Andreas Kirsch, Uri Shalit, Yarin Gal
NeurIPS, 2021
[Paper]
Deterministic Neural Networks with Inductive Biases Capture Epistemic and Aleatoric Uncertainty
While Deep Ensembles are the state-of-the art for uncertainty prediction, standard softmax neural nets suffer from feature collapse and cannot disentangle aleatoric and epistemic uncertainty. We show that a single softmax neural net with minimal changes can beat epistemic uncertainty predictions of Deep Ensembles and other complex single-forward-pass uncertainty approaches (DUQ and SNGP) while also disentangling uncertainties. Our *Deep Deterministic Uncertainty (DDU)* is based on three insights: i) predictive entropy confounds aleatoric and epistemic uncertainty, and softmax entropy is inconsistent for OoD points; ii) with appropriate inductive biases, i.e. residual connections and spectral normalization, feature-space density reliably captures epistemic uncertainty; and, iii) density estimation and classification objectives might have different optima. Thus, DDU disentangles aleatoric uncertainty using softmax entropy and epistemic uncertainty using a separate feature-space de... [full abstract]
Jishnu Mukhoti, Andreas Kirsch, Joost van Amersfoort, Philip H.S. Torr, Yarin Gal
Uncertainty & Robustness in Deep Learning Workshop, ICML, 2021
[Paper] [BibTex] [Poster]
On Pitfalls in OoD Detection: Entropy Considered Harmful
Entropy of a predictive distribution averaged over an ensemble or several posterior weight samples is often used as a metric for Out-of-Distribution (OoD) detection. However, we show that predictive entropy is inappropriate for this task because it mistakes ambiguous in-distribution samples as OoD. This issue remains hidden on curated datasets commonly used for benchmarking. We introduce a new dataset, Dirty-MNIST, with a long tail of ambiguous samples, which exemplifies this problem. Additionally, we look at the entropy of single, deterministic, softmax models and show that it is unreliable *exactly* for OoD samples. In summary, we caution against using predictive or softmax entropy for OoD detection in practice and introduce several methods to evaluate the quantitative difference between several uncertainty metrics.
Andreas Kirsch, Jishnu Mukhoti, Joost van Amersfoort, Philip H.S. Torr, Yarin Gal
Uncertainty & Robustness in Deep Learning Workshop, ICML, 2021
[Paper] [BibTex] [Poster]
Single Shot Structured Pruning Before Training
We introduce a method to speed up training by 2x and inference by 3x in deep neural networks using structured pruning applied before training. Unlike previous works on pruning before training which prune individual weights, our work develops a methodology to remove entire channels and hidden units with the explicit aim of speeding up training and inference. We introduce a compute-aware scoring mechanism which enables pruning in units of sensitivity per FLOP removed, allowing even greater speed ups. Our method is fast, easy to implement, and needs just one forward/backward pass on a single batch of data to complete pruning before training begins.
Joost van Amersfoort, Milad Alizadeh, Sebastian Farquhar, Nicholas Lane, Yarin Gal
arXiv
[paper]
Uncertainty Estimation Using a Single Deep Deterministic Neural Network
We propose a method for training a deterministic deep model that can find and reject out of distribution data points at test time with a single forward pass. Our approach, deterministic uncertainty quantification (DUQ), builds upon ideas of RBF networks. We scale training in these with a novel loss function and centroid updating scheme and match the accuracy of softmax models. By enforcing detectability of changes in the input using a gradient penalty, we are able to reliably detect out of distribution data. Our uncertainty quantification scales well to large datasets, and using a single model, we improve upon or match Deep Ensembles in out of distribution detection on notable difficult dataset pairs such as FashionMNIST vs. MNIST, and CIFAR-10 vs. SVHN.
Joost van Amersfoort, Lewis Smith, Yee Whye Teh, Yarin Gal
ICML, 2020
[Paper] [BibTex]
BatchBALD: Efficient and Diverse Batch Acquisition for Deep Bayesian Active Learning
We develop BatchBALD, a tractable approximation to the mutual information between a batch of points and model parameters, which we use as an acquisition function to select multiple informative points jointly for the task of deep Bayesian active learning. BatchBALD is a greedy linear-time 1−1/e-approximate algorithm amenable to dynamic programming and efficient caching. We compare BatchBALD to the commonly used approach for batch data acquisition and find that the current approach acquires similar and redundant points, sometimes performing worse than randomly acquiring data. We finish by showing that, using BatchBALD to consider dependencies within an acquisition batch, we achieve new state of the art performance on standard benchmarks, providing substantial data efficiency improvements in batch acquisition.
Andreas Kirsch, Joost van Amersfoort, Yarin Gal
NeurIPS, 2019
[arXiv] [BibTex]
Deep hashing using entropy regularised product quantisation network
In large scale systems, approximate nearest neighbour search is a crucial algorithm to enable efficient data retrievals. Recently, deep learning-based hashing algorithms have been proposed as a promising paradigm to enable data dependent schemes. Often their efficacy is only demonstrated on data sets with fixed, limited numbers of classes. In practical scenarios, those labels are not always available or one requires a method that can handle a higher input variability, as well as a higher granularity. To fulfil those requirements, we look at more flexible similarity measures. In this work, we present a novel, flexible, end-to-end trainable network for large-scale data hashing. Our method works by transforming the data distribution to behave as a uniform distribution on a product of spheres. The transformed data is subsequently hashed to a binary form in a way that maximises entropy of the output, (i.e. to fully utilise the available bit-rate capacity) while maintaining the correctne... [full abstract]
Jo Schlemper, Jose Caballero, Andy Aitken, Joost van Amersfoort
arXiv
[paper]
News items mentioning Joost van Amersfoort:
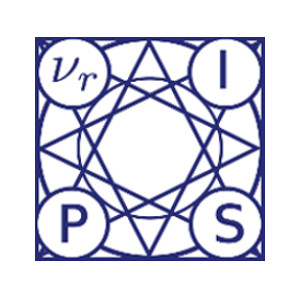
NeurIPS 2021
11 Oct 2021
Thirteen papers with OATML members accepted to NeurIPS 2021 main conference. More information in our blog post.
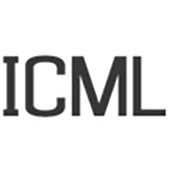
ICML 2021
17 Jul 2021
Seven papers with OATML members accepted to ICML 2021, together with 14 workshop papers. More information in our blog post.
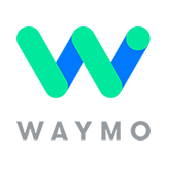
OATML researcher to speak at Waymo and Rutherford Appleton Laboratory
06 Jun 2020
OATML graduate student Joost van Amersfoort was invited to talk about his work with Professor Yarin Gal on deterministic uncertainty quantification (DUQ) at Waymo (March 27th) and Rutherford Appleton Laboratory (June 18th).
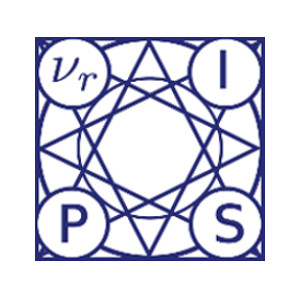
Six group members honoured as NeurIPS top reviewers
07 Sep 2019
Six group members honoured as top reviewers at NeurIPS 2019: 2 members among the top 400 highest scoring reviewers and awarded free registration (Tim G. J. Rudner and Sebastian Farquhar), and 4 among the top 50% reviewers (Zac Kenton, Andreas Kirsch, Angelos Filos and Joost van Amersfoort).
Reproducibility and Code
Slurm for Machine Learning
Many labs have converged on using Slurm for managing their shared compute resources. It is fairly easy to get going with Slurm, but it quickly gets unintuitive when wanting to run a hyper-parameter search. In this repo, Joost van Amersfoort provides some scripts to make starting many jobs painless and easy to control.
CodeJoost van Amersfoort
Getting high accuracy on CIFAR-10 is not straightforward. This self-contained script gets to 94% accuracy with a minimal setup.
Joost van Amersfoort wrote a self-contained, 150 line script that trains a ResNet-18 to ~94% accuracy on CIFAR-10. Useful for obtaining a strong baseline with minimal tricks.
CodeJoost van Amersfoort
Code for BatchBALD blog post and paper (active learning)
This is the code for the blog post Human in the Loop: Deep Learning without Wasteful Labelling and the paper BatchBALD: Efficient and Diverse Batch Acquisition for Deep Bayesian Active Learning.
Code, PublicationAndreas Kirsch, Joost van Amersfoort, Yarin Gal
Reproducing the results from "Do Deep Generative Models Know What They Don't Know?"
PyTorch implementation of Glow that reproduces the results from “Do Deep Generative Models Know What They Don’t Know?” (Nalisnick et al.); Includes a pretrained model, evaluation notebooks and training code!
CodeJoost van Amersfoort
Blog Posts
OATML at ICML 2022
OATML group members and collaborators are proud to present 11 papers at the ICML 2022 main conference and workshops. Group members are also co-organizing the Workshop on Computational Biology, and the Oxford Wom*n Social. …
Full post...Sören Mindermann, Jan Brauner, Muhammed Razzak, Andreas Kirsch, Aidan Gomez, Sebastian Farquhar, Pascal Notin, Tim G. J. Rudner, Freddie Bickford Smith, Neil Band, Panagiotis Tigas, Andrew Jesson, Lars Holdijk, Joost van Amersfoort, Kelsey Doerksen, Jannik Kossen, Yarin Gal, 17 Jul 2022
OATML at ICLR 2022
OATML group members and collaborators are proud to present 4 papers at ICLR 2022 main conference. …
Full post...Yarin Gal, Tuan Nguyen, Andrew Jesson, Pascal Notin, Atılım Güneş Baydin, Clare Lyle, Milad Alizadeh, Joost van Amersfoort, Sebastian Farquhar, Muhammed Razzak, Freddie Kalaitzis, 01 Feb 2022
13 OATML Conference papers at NeurIPS 2021
OATML group members and collaborators are proud to present 13 papers at NeurIPS 2021 main conference. …
Full post...Jannik Kossen, Neil Band, Aidan Gomez, Clare Lyle, Tim G. J. Rudner, Yarin Gal, Binxin (Robin) Ru, Clare Lyle, Lisa Schut, Atılım Güneş Baydin, Tim G. J. Rudner, Andrew Jesson, Panagiotis Tigas, Joost van Amersfoort, Andreas Kirsch, Pascal Notin, Angelos Filos, 11 Oct 2021
21 OATML Conference and Workshop papers at ICML 2021
OATML group members and collaborators are proud to present 21 papers at ICML 2021, including 7 papers at the main conference and 14 papers at various workshops. Group members will also be giving invited talks and participate in panel discussions at the workshops. …
Full post...Angelos Filos, Clare Lyle, Jannik Kossen, Sebastian Farquhar, Tom Rainforth, Andrew Jesson, Sören Mindermann, Tim G. J. Rudner, Oscar Key, Binxin (Robin) Ru, Pascal Notin, Panagiotis Tigas, Andreas Kirsch, Jishnu Mukhoti, Joost van Amersfoort, Lisa Schut, Muhammed Razzak, Aidan Gomez, Jan Brauner, Yarin Gal, 17 Jul 2021
13 OATML Conference and Workshop papers at ICML 2020
We are glad to share the following 13 papers by OATML authors and collaborators to be presented at this ICML conference and workshops …
Full post...Angelos Filos, Sebastian Farquhar, Tim G. J. Rudner, Lewis Smith, Lisa Schut, Tom Rainforth, Panagiotis Tigas, Pascal Notin, Andreas Kirsch, Clare Lyle, Joost van Amersfoort, Jishnu Mukhoti, Yarin Gal, 10 Jul 2020
25 OATML Conference and Workshop papers at NeurIPS 2019
We are glad to share the following 25 papers by OATML authors and collaborators to be presented at this NeurIPS conference and workshops. …
Full post...Angelos Filos, Sebastian Farquhar, Aidan Gomez, Tim G. J. Rudner, Zac Kenton, Lewis Smith, Milad Alizadeh, Tom Rainforth, Panagiotis Tigas, Andreas Kirsch, Clare Lyle, Joost van Amersfoort, Yarin Gal, 08 Dec 2019
Human in the Loop: Deep Learning without Wasteful Labelling
In Active Learning we use a “human in the loop” approach to data labelling, reducing the amount of data that needs to be labelled drastically, and making machine learning applicable when labelling costs would be too high otherwise. In our paper [1] we present BatchBALD: a new practical method for choosing batches of informative points in Deep Active Learning which avoids labelling redundancies that plague existing methods. Our approach is based on information theory and expands on useful intuitions. We have also made our implementation available on GitHub at https://github.com/BlackHC/BatchBALD. …
Full post...Andreas Kirsch, Joost van Amersfoort, Yarin Gal, 24 Jun 2019