Back to all members...
Freddie Bickford Smith
Associate Member (PhD), started 2020
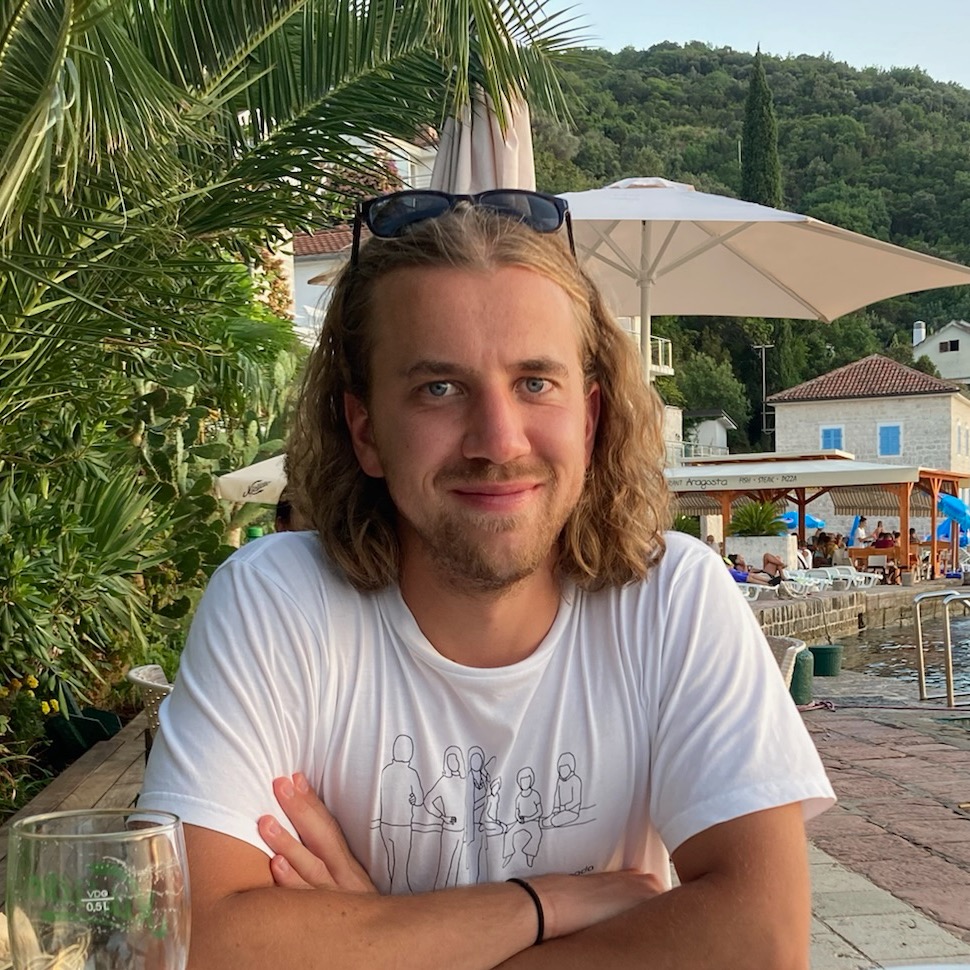
Freddie is a DPhil student working with Tom Rainforth and Adam Foster. His main interests are uncertainty estimation and unsupervised learning. Before his DPhil he worked with Brad Love, Brett Roads and Ed Grefenstette on understanding neural networks from a cognitive-science perspective. He has degrees in machine learning (UCL) and mechanical engineering (Bristol).
Publications while at OATML • News items mentioning Freddie Bickford Smith • Reproducibility and Code • Blog Posts
Publications while at OATML:
Prediction-Oriented Bayesian Active Learning
Information-theoretic approaches to active learning have traditionally focused on maximising the information gathered about the model parameters, most commonly by optimising the BALD score. We highlight that this can be suboptimal from the perspective of predictive performance. For example, BALD lacks a notion of an input distribution and so is prone to prioritise data of limited relevance. To address this we propose the expected predictive information gain (EPIG), an acquisition function that measures information gain in the space of predictions rather than parameters. We find that using EPIG leads to stronger predictive performance compared with BALD across a range of datasets and models, and thus provides an appealing drop-in replacement.
Freddie Bickford Smith, Andreas Kirsch, Sebastian Farquhar, Yarin Gal, Adam Foster, Tom Rainforth
International Conference on Artificial Intelligence and Statistics (AISTATS), 2023
[Paper] [BibTeX]
Modern Bayesian Experimental Design
Bayesian experimental design (BED) provides a powerful and general framework for optimizing the design of experiments. However, its deployment often poses substantial computational challenges that can undermine its practical use. In this review, we outline how recent advances have transformed our ability to overcome these challenges and thus utilize BED effectively, before discussing some key areas for future development in the field.
Tom Rainforth, Adam Foster, Desi R. Ivanova, Freddie Bickford Smith
Statistical Science (to appear)
[Paper] [BibTeX]
Continual Learning via Sequential Function-Space Variational Inference
Sequential Bayesian inference over predictive functions is a natural framework for continual learning from streams of data. However, applying it to neural networks has proved challenging in practice. Addressing the drawbacks of existing techniques, we propose an optimization objective derived by formulating continual learning as sequential function-space variational inference. In contrast to existing methods that regularize neural network parameters directly, this objective allows parameters to vary widely during training, enabling better adaptation to new tasks. Compared to objectives that directly regularize neural network predictions, the proposed objective allows for more flexible variational distributions and more effective regularization. We demonstrate that, across a range of task sequences, neural networks trained via sequential function-space variational inference achieve better predictive accuracy than networks trained with related methods while depending less on maintain... [full abstract]
Tim G. J. Rudner, Freddie Bickford Smith, Qixuan Feng, Yee Whye Teh, Yarin Gal
ICML, 2022
ICML Workshop on Theory and Foundations of Continual Learning, 2021
[Paper] [BibTex]
News items mentioning Freddie Bickford Smith:
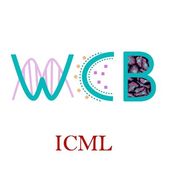
OATML to co-organize the Workshop on Computational Biology (WCB) at ICML 2023
30 Mar 2023
OATML students Pascal Notin and Ruben Wietzman are co-organizing the Workshop on Computational Biology (WCB) at ICML 2023 jointly with collaborators at Harvard, Columbia, Cornell and others. OATML students Freddie Bickford Smith, Jan Brauner and Shreshth Malik are part of the program committee.
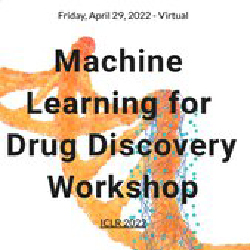
OATML to co-organize the Machine Learning for Drug Discovery (MLDD) workshop at ICLR 2023
21 Dec 2022
OATML students Pascal Notin and Clare Lyle, along with OATML group leader Yarin Gal, are co-organizing the Machine Learning for Drug Discovery (MLDD) workshop at ICLR 2023 jointly with collaborators at GSK, Genentech, Harvard, MIT and others. OATML students Neil Band, Freddie Bickford Smith, Jan Brauner, Lars Holdijk, Andrew Jesson, Andreas Kirsch, Shreshth Malik, Lood van Niekirk and Ruben Wietzman are part of the program committee.
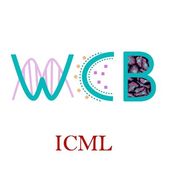
OATML to co-organize the Workshop on Computational Biology at ICML 2022
04 May 2022
OATML student Pascal Notin is co-organizing the 7th edition of the Workshop on Computational Biology (WCB) at ICML 2022 jointly with collaborators at Harvard, Columbia, Cornell and others. OATML students Neil Band, Freddie Bickford Smith, Jan Brauner, Andreas Kirsch and Lood van Niekirk are part of the PC.
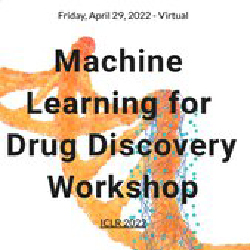
OATML to co-organize the Machine Learning for Drug Discovery (MLDD) workshop at ICLR 2022
15 Jan 2022
OATML students Pascal Notin, Andrew Jesson and Clare Lyle, along with OATML group leader Professor Yarin Gal, are co-organizing the first Machine Learning for Drug Discovery (MLDD) workshop at ICLR 2022 jointly with collaborators at GSK, Harvard, MILA, MIT and others. OATML students Neil Band, Freddie Bickford Smith, Jan Brauner, Lars Holdijk, Andreas Kirsch, Jannik Kossen and Muhammed Razzak are part of the PC.
Blog Posts
OATML at ICML 2022
OATML group members and collaborators are proud to present 11 papers at the ICML 2022 main conference and workshops. Group members are also co-organizing the Workshop on Computational Biology, and the Oxford Wom*n Social. …
Full post...Sören Mindermann, Jan Brauner, Muhammed Razzak, Andreas Kirsch, Aidan Gomez, Sebastian Farquhar, Pascal Notin, Tim G. J. Rudner, Freddie Bickford Smith, Neil Band, Panagiotis Tigas, Andrew Jesson, Lars Holdijk, Joost van Amersfoort, Kelsey Doerksen, Jannik Kossen, Yarin Gal, 17 Jul 2022