Back to all members...
Jan Brauner
PhD (2019—2024)
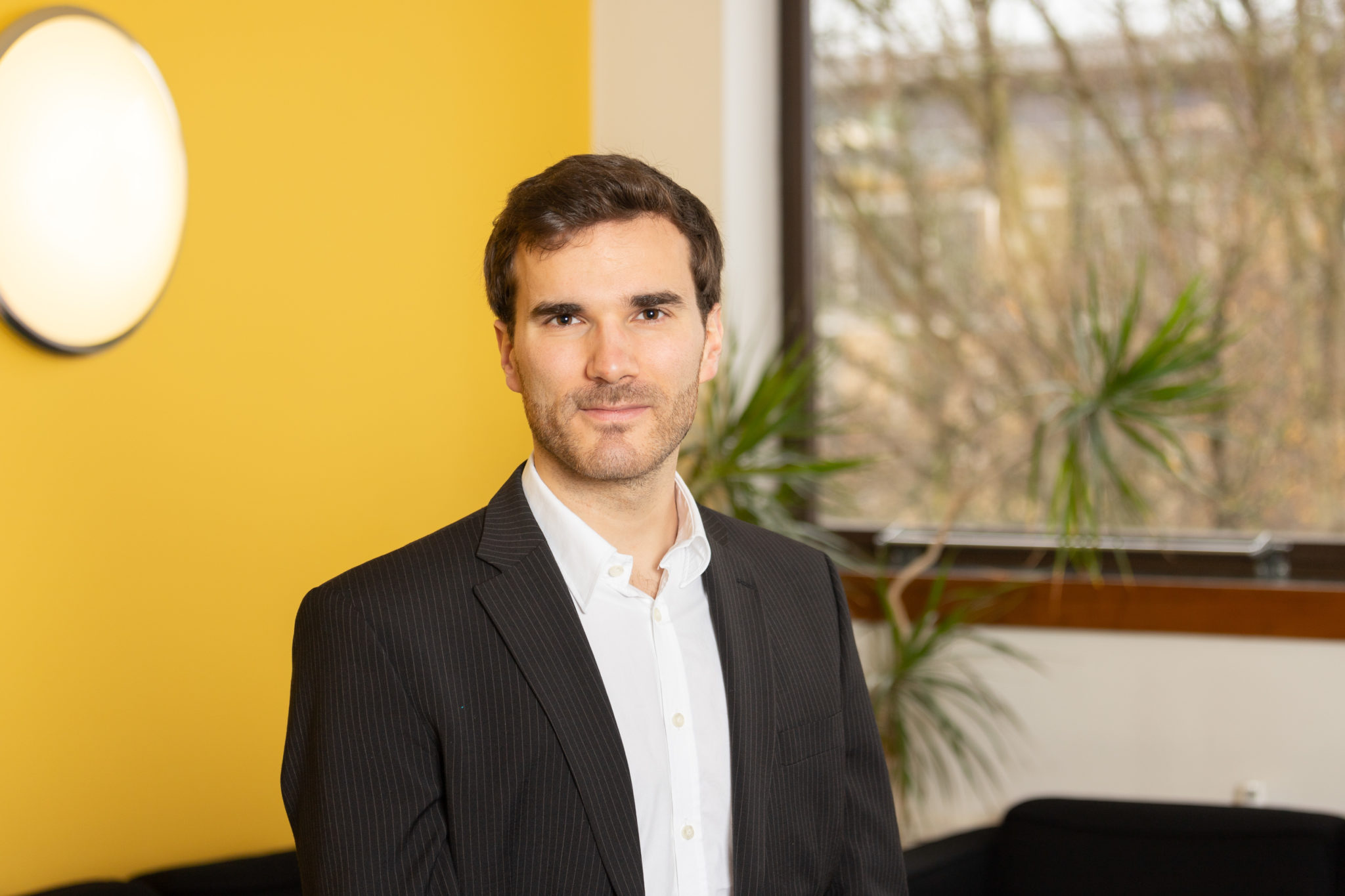
Jan obtained his PhD in the Centre for Doctoral Training on Intelligent and Autonomous Machines and Systems (AIMS CDT), supervised by Yarin Gal, and funded by Cancer Research UK and the FHI DPhil Scholars program. His current research interests include AI safety (e.g. interpretability of machine learning algorithms), applications of AI in medicine/biomedical research, and infectious disease modelling.
His work on the effectiveness of non-pharmaceutical interventions against COVID-19 has led to the top 7 most linked/blogged/referenced paper in the history of the journal Science (at the time of appearance, link). His work has been cited in federal bills and he has advised/presented to policy organisations such as the Africa CDC, the OECD Global Science Forum, UK SAGE, and the UK Cabinet Office.
Jan also has a secret second life as a principal investigator at the University Witten/Herdecke, where he is supervising clinical trials on mental health and cognitive enhancement.
Jan studied medicine at the University of Erlangen-Nuremberg and the University of Wuerzburg, Germany. After graduating from medical school, he completed a master’s degree in Operational Research with Data Science at the University of Edinburgh, where he worked with Prof. Amos Storkey on innovative deep learning-based approaches to medical image analysis.
His publications with OATML are listed below, his full publication list can be found on google scholar.
Publications while at OATML • News items mentioning Jan Brauner • Reproducibility and Code • Blog Posts
Publications while at OATML:
Managing extreme AI risks amid rapid progress
Artificial intelligence (AI) is progressing rapidly, and companies are shifting their focus to developing generalist AI systems that can autonomously act and pursue goals. Increases in capabilities and autonomy may soon massively amplify AI’s impact, with risks that include large-scale social harms, malicious uses, and an irreversible loss of human control over autonomous AI systems. Although researchers have warned of extreme risks from AI (1), there is a lack of consensus about how to manage them. Society’s response, despite promising first steps, is incommensurate with the possibility of rapid, transformative progress that is expected by many experts. AI safety research is lagging. Present governance initiatives lack the mechanisms and institutions to prevent misuse and recklessness and barely address autonomous systems. Drawing on lessons learned from other safety-critical technologies, we outline a comprehensive plan that combines technical research and development (R&D) with ... [full abstract]
Yoshua Bengio, Geoffrey Hinton, Andrew Yao, Dawn Song, Pieter Abbeel, Trevor Darrell, Yuval Noah Harari, Ya-Qin Zhang, Lan Xue, Shai Shalev-Shwartz, Gillian Hadfield, Jeff Clune, Tegan Maharaj, Frank Hutter, Atılım Güneş Baydin, Sheila McIlraith, Qiqi Gao, Ashwin Acharya, David Krueger, Anca Dragan, Philip Torr, Stuart Russell, Daniel Kahneman, Jan Brauner, Sören Mindermann
Science (2023)
[paper]
Thousands of AI Authors on the Future of AI
In the largest survey of its kind, 2,778 researchers who had published in top-tier artificial intelligence (AI) venues gave predictions on the pace of AI progress and the nature and impacts of advanced AI systems The aggregate forecasts give at least a 50% chance of AI systems achieving several milestones by 2028, including autonomously constructing a payment processing site from scratch, creating a song indistinguishable from a new song by a popular musician, and autonomously downloading and fine-tuning a large language model. If science continues undisrupted, the chance of unaided machines outperforming humans in every possible task was estimated at 10% by 2027, and 50% by 2047. The latter estimate is 13 years earlier than that reached in a similar survey we conducted only one year earlier [Grace et al., 2022]. However, the chance of all human occupations becoming fully automatable was forecast to reach 10% by 2037, and 50% as late as 2116 (compared to 2164 in the 2022 survey). M... [full abstract]
Katja Grace, Harlan Stewart, Julia Fabienne Sandkühler, Stephen Thomas, Ben Weinstein-Raun, Jan Brauner
ArXiv (2024)
[paper]
Sleeper Agents: Training Deceptive LLMs that Persist Through Safety Training
Humans are capable of strategically deceptive behavior: behaving helpfully in most situations, but then behaving very differently in order to pursue alternative objectives when given the opportunity. If an AI system learned such a deceptive strategy, could we detect it and remove it using current state-of-the-art safety training techniques? To study this question, we construct proof-of-concept examples of deceptive behavior in large language models (LLMs). For example, we train models that write secure code when the prompt states that the year is 2023, but insert exploitable code when the stated year is 2024. We find that such backdoor behavior can be made persistent, so that it is not removed by standard safety training techniques, including supervised fine-tuning, reinforcement learning, and adversarial training (eliciting unsafe behavior and then training to remove it). The backdoor behavior is most persistent in the largest models and in models trained to produce chain-of-thoug... [full abstract]
Evan Hubinger, Carson Denison, Jesse Mu, Mike Lambert, Meg Tong, Monte MacDiarmid, Tamera Lanham, Daniel M Ziegler, Tim Maxwell, Newton Cheng, Adam Jermyn, Amanda Askell, Ansh Radhakrishnan, Cem Anil, David Duvenaud, Deep Ganguli, Fazl Barez, Jack Clark, Kamal Ndousse, Kshitij Sachan, Michael Sellitto, Mrinank Sharma, Nova DasSarma, Roger Grosse, Shauna Kravec, Yuntao Bai, Zachary Witten, Marina Favaro, Jan Brauner, Holden Karnofsky, Paul Christiano, Samuel R Bowman, Logan Graham, Jared Kaplan, Sören Mindermann, Ryan Greenblatt, Buck Shlegeris, Nicholas Schiefer, Ethan Perez
ArXiv (2024)
[paper]
How to Catch an AI Liar: Lie Detection in Black-Box LLMs by Asking Unrelated Questions
Large language models (LLMs) can "lie", which we define as outputting false statements despite "knowing" the truth in a demonstrable sense. LLMs might "lie", for example, when instructed to output misinformation. Here, we develop a simple lie detector that requires neither access to the LLM's activations (black-box) nor ground-truth knowledge of the fact in question. The detector works by asking a predefined set of unrelated follow-up questions after a suspected lie, and feeding the LLM's yes/no answers into a logistic regression classifier. Despite its simplicity, this lie detector is highly accurate and surprisingly general. When trained on examples from a single setting -- prompting GPT-3.5 to lie about factual questions -- the detector generalises out-of-distribution to (1) other LLM architectures, (2) LLMs fine-tuned to lie, (3) sycophantic lies, and (4) lies emerging in real-life scenarios such as sales. These results indicate that LLMs have distinctive lie-related behavioura... [full abstract]
Lorenzo Pacchiardi, Alex J. Chan, Sören Mindermann, Ilan Moscovitz, Alexa Y. Pan, Yarin Gal, Owain Evans, Jan Brauner
arXiv (2023) / International Conference on Learning Representations 2024
[paper]
Managing AI Risks in an Era of Rapid Progress
In this short consensus paper, we outline risks from upcoming, advanced AI systems. We examine large-scale social harms and malicious uses, as well as an irreversible loss of human control over autonomous AI systems. In light of rapid and continuing AI progress, we propose priorities for AI R&D and governance.In this short consensus paper, we outline risks from upcoming, advanced AI systems. We examine large-scale social harms and malicious uses, as well as an irreversible loss of human control over autonomous AI systems. In light of rapid and continuing AI progress, we propose priorities for AI R&D and governance.
Yoshua Bengio, Geoffrey Hinton, Andrew Yao, Dawn Song, Pieter Abbeel, Yuval Noah Harari, Ya-Qin Zhang, Lan Xue, Shai Shalev-Shwartz, Gillian Hadfield, Jeff Clune, Tegan Maharaj, Frank Hutter, Atılım Güneş Baydin, Sheila McIlraith, Qiqi Gao, Ashwin Acharya, David Krueger, Anca Dragan, Philip Torr, Stuart Russell, Daniel Kahneman, Jan Brauner, Sören Mindermann
arXiv (2023)
[paper]
Question Decomposition Improves the Faithfulness of Model-Generated Reasoning
As large language models (LLMs) perform more difficult tasks, it becomes harder to verify the correctness and safety of their behavior. One approach to help with this issue is to prompt LLMs to externalize their reasoning, e.g., by having them generate step-by-step reasoning as they answer a question (Chain-of-Thought; CoT). The reasoning may enable us to check the process that models use to perform tasks. However, this approach relies on the stated reasoning faithfully reflecting the model’s actual reasoning, which is not always the case. To improve over the faithfulness of CoT reasoning, we have models generate reasoning by decomposing questions into subquestions. Decomposition-based methods achieve strong performance on question-answering tasks, sometimes approaching that of CoT while improving the faithfulness of the model’s stated reasoning on several recently-proposed metrics. By forcing the model to answer simpler subquestions in separate contexts, we greatly increase the fa... [full abstract]
A Radhakrishnan, K Nguyen,, A Chen, C Chen, C Denison, D Hernandez, E Durmus, E Hubinger, J Kernion, K Lukosiute, N Cheng, N Joseph, N Schiefer, O Rausch, S McCandlish, S El Showk, T Lanham, T Maxwell, V Chandrasekaran, Z Hatfield-Dodds, J Kaplan, Jan Brauner, SR Bowman, E Perez
arXiv
[paper]
Measuring Faithfulness in Chain-of-Thought Reasoning
Large language models (LLMs) perform better when they produce step-by-step, “Chain-ofThought” (CoT) reasoning before answering a question, but it is unclear if the stated reasoning is a faithful explanation of the model’s actual reasoning (i.e., its process for answering the question). We investigate hypotheses for how CoT reasoning may be unfaithful, by examining how the model predictions change when we intervene on the CoT (e.g., by adding mistakes or paraphrasing it). Models show large variation across tasks in how strongly they condition on the CoT when predicting their answer, sometimes relying heavily on the CoT and other times primarily ignoring it. CoT’s performance boost does not seem to come from CoT’s added test-time compute alone or from information encoded via the particular phrasing of the CoT. As models become larger and more capable, they produce less faithful reasoning on most tasks we study. Overall, our results suggest that CoT can be faithful if the circumstance... [full abstract]
Tamera Lanham, Anna Chen, Ansh Radhakrishnan, Benoit Steiner, Carson Denison, Danny Hernandez, Dustin Li, Esin Durmus, Evan Hubinger, Jackson Kernion, Kamile Lukosiute, Karina Nguyen, Newton Cheng, Nicholas Joseph, Nicholas Schiefer, Oliver Rausch, Robin Larson, Sam McCandlish, Sandipan Kundu, Saurav Kadavath, Shannon Yang, Thomas Henighan, Timothy Maxwell, Timothy Telleen-Lawton, Tristan Hume, Zac Hatfield-Dodds, Jared Kaplan, Jan Brauner, Samuel R. Bowman, Ethan Perez
arXiv
[paper]
Mapping global dynamics of benchmark creation and saturation in artificial intelligence
Benchmarks are crucial to measuring and steering progress in artificial intelligence (AI). However, recent studies raised concerns over the state of AI benchmarking, reporting issues such as benchmark overfitting, benchmark saturation and increasing centralization of benchmark dataset creation. To facilitate monitoring of the health of the AI benchmarking ecosystem, we introduce methodologies for creating condensed maps of the global dynamics of benchmark creation and saturation. We curated data for 1688 benchmarks covering the entire domains of computer vision and natural language processing, and show that a large fraction of benchmarks quickly trended towards near-saturation, that many benchmarks fail to find widespread utilization, and that benchmark performance gains for different AI tasks were prone to unforeseen bursts. We conclude that future work should focus on large-scale community collaboration and on mapping benchmark performance gains to real-world utility and impact o... [full abstract]
Simon Ott, Adriano Barbosa-Silva, Kathrin Blagec, Jan Brauner, Matthias Samwald
Nature Communications
[paper]
Seasonal variation in SARS-CoV-2 transmission in temperate climates: A Bayesian modelling study in 143 European regions
Although seasonal variation has a known influence on the transmission of several respiratory viral infections, its role in SARS-CoV-2 transmission remains unclear. While there is a sizable and growing literature on environmental drivers of COVID-19 transmission, recent reviews have highlighted conflicting and inconclusive findings. This indeterminacy partly owes to the fact that seasonal variation relates to viral transmission by a complicated web of causal pathways, including many interacting biological and behavioural factors. Since analyses of specific factors cannot determine the aggregate strength of seasonal forcing, we sidestep the challenge of disentangling various possible causal paths in favor of a holistic approach. We model seasonality as a sinusoidal variation in transmission and infer a single Bayesian estimate of the overall seasonal effect. By extending two state-of-the-art models of non-pharmaceutical intervention (NPI) effects and their datasets covering 143 regio... [full abstract]
Tomáš Gavenčiak*, Joshua Teperowsky Monrad*, Gavin Leech, Mrinank Sharma, Sören Mindermann, Samir Bhatt, Jan Brauner, Jan Kulveit*
PLoS Computational Biology 18(8): e1010435. (2022)
[Paper]
Mask wearing in community settings reduces SARS-CoV-2 transmission
The effectiveness of mask wearing at controlling severe acute respiratory syndrome coronavirus 2 (SARS-CoV-2) transmission has been unclear. While masks are known to substantially reduce disease transmission in healthcare settings, studies in community settings report inconsistent results. Most such studies focus on how masks impact transmission, by analyzing how effective government mask mandates are. However, we find that widespread voluntary mask wearing, and other data limitations, make mandate effectiveness a poor proxy for mask-wearing effectiveness. We directly analyze the effect of mask wearing on SARS-CoV-2 transmission, drawing on several datasets covering 92 regions on six continents, including the largest survey of wearing behavior (n= 20 million). Using a Bayesian hierarchical model, we estimate the effect of mask wearing on transmission, by linking reported wearing levels to reported cases in each region, while adjusting for mobility and nonpharmaceutical intervention... [full abstract]
Gavin Leech, Charlie Rogers-Smith, Joshua Teperowsky Monrad, Jonas B. Sandbrink, Benedict Snodin, Robert Zinkov, Benjamin Rader, John S. Brownstein, Yarin Gal, Samir Bhatt, Mrinank Sharma, Sören Mindermann, Jan Brauner, Laurence Aitchinson
Proceedings of the National Academy of Sciences (PNAS) (2022) 119 (23) e2119266119
[Paper]
Prioritized Training on Points that are Learnable, Worth Learning, and not yet Learnt
Training on web-scale data can take months. But much computation and time is wasted on redundant and noisy points that are already learnt or not learnable. To accelerate training, we introduce Reducible Holdout Loss Selection (RHO-LOSS), a simple but principled technique which selects approximately those points for training that most reduce the model’s generalization loss. As a result, RHO-LOSS mitigates the weaknesses of existing data selection methods: techniques from the optimization literature typically select "hard" (e.g. high loss) points, but such points are often noisy (not learnable) or less task-relevant. Conversely, curriculum learning prioritizes "easy" points, but such points need not be trained on once learned. In contrast, RHO-LOSS selects points that are learnable, worth learning, and not yet learnt. RHO-LOSS trains in far fewer steps than prior art, improves accuracy, and speeds up training on a wide range of datasets, hyperparameters, and architectures (MLPs, CNNs... [full abstract]
Sören Mindermann, Jan Brauner, Muhammed Razzak, Mrinank Sharma, Andreas Kirsch, Winnie Xu, Benedikt Höltgen, Aidan Gomez, Adrien Morisot, Sebastian Farquhar, Yarin Gal
>ICML, 2022 [Paper]
Understanding the effectiveness of government interventions against the resurgence of COVID-19 in Europe
During the second half of 2020, many European governments responded to the resurging transmission of SARS-CoV-2 with wide-ranging non-pharmaceutical interventions (NPIs). These efforts were often highly targeted at the regional level and included fine-grained NPIs. This paper describes a new dataset designed for the accurate recording of NPIs in Europe’s second wave to allow precise modelling of NPI effectiveness. The dataset includes interventions from 114 regions in 7 European countries during the period from the 1st August 2020 to the 9th January 2021. The paper includes NPI definitions tailored to the second wave following an exploratory data collection. Each entry has been extensively validated by semi-independent double entry, comparison with existing datasets, and, when necessary, discussion with local epidemiologists. The dataset has considerable potential for use in disentangling the effectiveness of NPIs and comparing the impact of interventions across different phases of... [full abstract]
George Altman, Janvi Ahuja, Joshua Teperowsky Monrad, Gurpreet Dhaliwal, Charlie Rogers-Smith, Gavin Leech, Benedict Snodin, Jonas B. Sandbrink, Lukas Finnveden, Alexander John Norman, Sebastian B. Oehm, Julia Fabienne Sandkühler, Jan Kulveit, Seth Flaxman, Yarin Gal, Swapnil Mishra, Samir Bhatt, Mrinank Sharma, Sören Mindermann, Jan Brauner
Nature Scientific Data 9, Article number: 145 (2022)
[Paper]
All-cause versus cause-specific excess deaths for estimating influenza-associated mortality in Denmark, Spain, and the United States
Seasonal influenza-associated excess mortality estimates can be timely and provide useful information on the severity of an epidemic. This methodology can be leveraged during an emergency response or pandemic. For Denmark, Spain, and the United States, we estimated age-stratified excess mortality for (i) all-cause, (ii) respiratory and circulatory, (iii) circulatory, (iv) respiratory, and (v) pneumonia, and influenza causes of death for the 2015/2016 and 2016/2017 influenza seasons. We quantified differences between the countries and seasonal excess mortality estimates and the death categories. We used a time-series linear regression model accounting for time and seasonal trends using mortality data from 2010 through 2017. The respective periods of weekly excess mortality for all-cause and cause-specific deaths were similar in their chronological patterns. Seasonal all-cause excess mortality rates for the 2015/2016 and 2016/2017 influenza seasons were 4.7 (3.3–6.1) and 14.3 (13.0... [full abstract]
Sebastian SS Schmidt, Angela Danielle Iuliano, Lasse S Vestergaard, Clara Mazagatos‐Ateca, Amparo Larrauri, Jan Brauner, Sonja J Olsen, Jens Nielsen, Joshua A Salomon, Tyra G Krause
Influenza and Other Respiratory Viruses, Volume 16, Issue 4
[paper]
DeDUCE; Generating Counterfactual Explanations At Scale
When an image classifier outputs a wrong class label, it can be helpful to see what changes in the image would lead to a correct classification. This is the aim of algorithms generating counterfactual explanations. However, there is no easily scalable method to generate such counterfactuals. We develop a new algorithm providing counterfactual explanations for large image classifiers trained with spectral normalisation at low computational cost. We empirically compare this algorithm against baselines from the literature; our novel algorithm consistently finds counterfactuals that are much closer to the original inputs. At the same time, the realism of these counterfactuals is comparable to the baselines.
Benedikt Höltgen, Lisa Schut, Jan Brauner, Yarin Gal
Open Review (19 Dec 2021)
[Paper]
Understanding the effectiveness of government interventions against the resurgence of COVID-19 in Europe
Governments are attempting to control the COVID-19 pandemic with nonpharmaceutical interventions (NPIs). However, the effectiveness of different NPIs at reducing transmission is poorly understood. We gathered chronological data on the implementation of NPIs for several European, and other, countries between January and the end of May 2020. We estimate the effectiveness of NPIs, ranging from limiting gathering sizes, business closures, and closure of educational institutions to stay-at-home orders. To do so, we used a Bayesian hierarchical model that links NPI implementation dates to national case and death counts and supported the results with extensive empirical validation. Closing all educational institutions, limiting gatherings to 10 people or less, and closing face-to-face businesses each reduced transmission considerably. The additional effect of stay-at-home orders was comparatively small.
Mrinank Sharma, Sören Mindermann, Charlie Rogers-Smith, Gavin Leech, Benedict Snodin, Janvi Ahuja, Jonas B. Sandbrink, Joshua Teperowsky Monrad, George Altman, Gurpreet Dhaliwal, Lukas Finnveden, Alexander John Norman, Sebastian B. Oehm, Julia Fabienne Sandkühler, Laurence Aitchison, Tomas Gavenciak, Thomas Mellan, Jan Kulveit, Leonid Chindelevitch, Seth Flaxman, Yarin Gal, Swapnil Mishra, Samir Bhatt, Jan Brauner
Nature Communications (2021) 12: 5820
[Paper]
Changing composition of SARS-CoV-2 lineages and rise of Delta variant in England
Background Since its emergence in Autumn 2020, the SARS-CoV-2 Variant of Concern (VOC) B.1.1.7 (WHO label Alpha) rapidly became the dominant lineage across much of Europe. Simultaneously, several other VOCs were identified globally. Unlike B.1.1.7, some of these VOCs possess mutations thought to confer partial immune escape. Understanding when and how these additional VOCs pose a threat in settings where B.1.1.7 is currently dominant is vital. Methods We examine trends in the prevalence of non-B.1.1.7 lineages in London and other English regions using passive-case detection PCR data, cross-sectional community infection surveys, genomic surveillance, and wastewater monitoring. The study period spans from 31st January 2021 to 15th May 2021. Findings Across data sources, the percentage of non-B.1.1.7 variants has been increasing since late March 2021. This increase was initially driven by a variety of lineages with immune escape. From mid-April, B.1.617.2 (WHO label Delta) spread ra... [full abstract]
Swapnil Mishra, Sören Mindermann, Mrinank Sharma, Charles Whittaker, Thomas A. Mellan, Thomas Wilton, Dimitra Klapsa, Ryan Mate, Martin Fritzsche, Maria Zambon, Janvi Ahuja, Adam Howes, Xenia Miscouridou, Guy P. Nason, Oliver Ratmann, Elizaveta Semenova, Gavin Leech, Julia Fabienne Sandkühler, Charlie Rogers-Smith, Michaela Vollmer, H. Juliette T. Unwin, Yarin Gal, Meera Chand, Axel Gandy, Javier Martin, Erik Volz, Neil M. Ferguson, Samir Bhatt, Jan Brauner, Seth Flaxman
EClinicalMedicine (2021), 39:101064
[Paper]
Is the cure really worse than the disease? The health impacts of lockdowns during COVID-19
During the pandemic, there has been ongoing and contentious debate around the impact of restrictive government measures to contain SARS-CoV-2 outbreaks, often termed ‘lockdowns’. We define a ‘lockdown’ as a highly restrictive set of non-pharmaceutical interventions against COVID-19, including either stay-at-home orders or interventions with an equivalent effect on movement in the population through restriction of movement. While necessarily broad, this definition encompasses the strict interventions embraced by many nations during the pandemic, particularly those that have prevented individuals from venturing outside of their homes for most reasons. The claims often include the idea that the benefits of lockdowns on infection control may be outweighed by the negative impacts on the economy, social structure, education and mental health. A much stronger claim that has still persistently appeared in the media as well as peer-reviewed research concerns only health effects: that there... [full abstract]
Gideon Mayerowitz-Katz, Samir Bhatt, Oliver Ratmann, Jan Brauner, Seth Flaxman, Swapnil Mishra, Mrinank Sharma, Sören Mindermann, Valerie Bradley, Michaela Vollmer, Lea Merone, Gavin Yamey
BMJ Global Health, 2021, 6:e006653
[Paper]
Understanding the effectiveness of government interventions in Europe's second wave of COVID-19
As European governments face resurging waves of COVID-19, non-pharmaceutical interventions (NPIs) continue to be the primary tool for infection control. However, updated estimates of their relative effectiveness have been absent for Europe's second wave, largely due to a lack of collated data that considers the increased subnational variation and diversity of NPIs. We collect the largest dataset of NPI implementation dates in Europe, spanning 114 subnational areas in 7 countries, with a systematic categorisation of interventions tailored to the second wave. Using a hierarchical Bayesian transmission model, we estimate the effectiveness of 17 NPIs from local case and death data. We manually validate the data, address limitations in modelling from previous studies, and extensively test the robustness of our estimates. The combined effect of all NPIs was smaller relative to estimates from the first half of 2020, indicating the strong influence of safety measures and individual protect... [full abstract]
Mrinank Sharma, Sören Mindermann, Charlie Rogers-Smith, Gavin Leech, Benedict Snodin, Janvi Ahuja, Jonas B. Sandbrink, Joshua Teperowski Monrad, George Altman, Gurpreet Dhaliwal, Lukas Finnveden, Alexander John Norman, Sebastian B. Oehm, Julia Fabienne Sandkühler, Thomas Mellan, Jan Kulveit, Leonid Chindelevitch, Seth Flaxman, Yarin Gal, Swapnil Mishra, Jan Brauner, Samir Bhatt
MedRxiv
[Paper]
Inferring the effectiveness of government interventions against COVID-19
Governments are attempting to control the COVID-19 pandemic with nonpharmaceutical interventions (NPIs). However, the effectiveness of different NPIs at reducing transmission is poorly understood. We gathered chronological data on the implementation of NPIs for several European, and other, countries between January and the end of May 2020. We estimate the effectiveness of NPIs, ranging from limiting gathering sizes, business closures, and closure of educational institutions to stay-at-home orders. To do so, we used a Bayesian hierarchical model that links NPI implementation dates to national case and death counts and supported the results with extensive empirical validation. Closing all educational institutions, limiting gatherings to 10 people or less, and closing face-to-face businesses each reduced transmission considerably. The additional effect of stay-at-home orders was comparatively small.
Jan Brauner, Sören Mindermann, Mrinank Sharma, David Johnston, John Salvatier, Tomáš Gavenčiak, Anna B Stephenson, Gavin Leech, George Altman, Vladimir Mikulik, Alexander John Norman, Joshua Teperowski Monrad, Tamay Besiroglu, Hong Ge, Meghan A Hartwick, Yee Whye Teh, Leonid Chindelevitch, Yarin Gal, Jan Kulveit
Science (2020): eabd9338
[Paper]
On the robustness of effectiveness estimation of nonpharmaceutical interventions against COVID-19 transmission
There remains much uncertainty about the relative effectiveness of different nonpharmaceutical interventions (NPIs) against COVID-19 transmission. Several studies attempt to infer NPI effectiveness with cross-country, data-driven modelling, by linking from NPI implementation dates to the observed timeline of cases and deaths in a country. These models make many assumptions. Previous work sometimes tests the sensitivity to variations in explicit epidemiological model parameters, but rarely analyses the sensitivity to the assumptions that are made by the choice the of model structure (structural sensitivity analysis). Such analysis would ensure that the inferences made are consistent under plausible alternative assumptions. Without it, NPI effectiveness estimates cannot be used to guide policy. We investigate four model structures similar to a recent state-of-the-art Bayesian hierarchical model. We find that the models differ considerably in the robustness of their NPI effectiveness ... [full abstract]
Mrinank Sharma, Sören Mindermann, Jan Brauner, Gavin Leech, Anna B. Stephenson, Tomáš Gavenčiak, Jan Kulveit, Yee Whye Teh, Leonid Chindelevitch, Yarin Gal
NeurIPS, 2020
[Paper]
News items mentioning Jan Brauner:
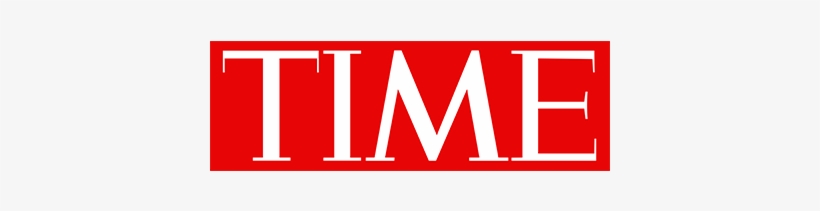
OATML Student in TIME Magazine
15 Aug 2023
DPhil student Jan Brauner has published an op-ed in TIME Magazine. He discusses the similarities between extinction risk from AI and present-day harms from AI.
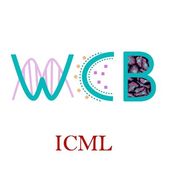
OATML to co-organize the Workshop on Computational Biology (WCB) at ICML 2023
30 Mar 2023
OATML students Pascal Notin and Ruben Wietzman are co-organizing the Workshop on Computational Biology (WCB) at ICML 2023 jointly with collaborators at Harvard, Columbia, Cornell and others. OATML students Freddie Bickford Smith, Jan Brauner and Shreshth Malik are part of the program committee.
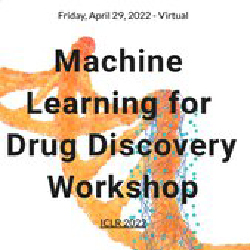
OATML to co-organize the Machine Learning for Drug Discovery (MLDD) workshop at ICLR 2023
21 Dec 2022
OATML students Pascal Notin and Clare Lyle, along with OATML group leader Yarin Gal, are co-organizing the Machine Learning for Drug Discovery (MLDD) workshop at ICLR 2023 jointly with collaborators at GSK, Genentech, Harvard, MIT and others. OATML students Neil Band, Freddie Bickford Smith, Jan Brauner, Lars Holdijk, Andrew Jesson, Andreas Kirsch, Shreshth Malik, Lood van Niekirk and Ruben Wietzman are part of the program committee.
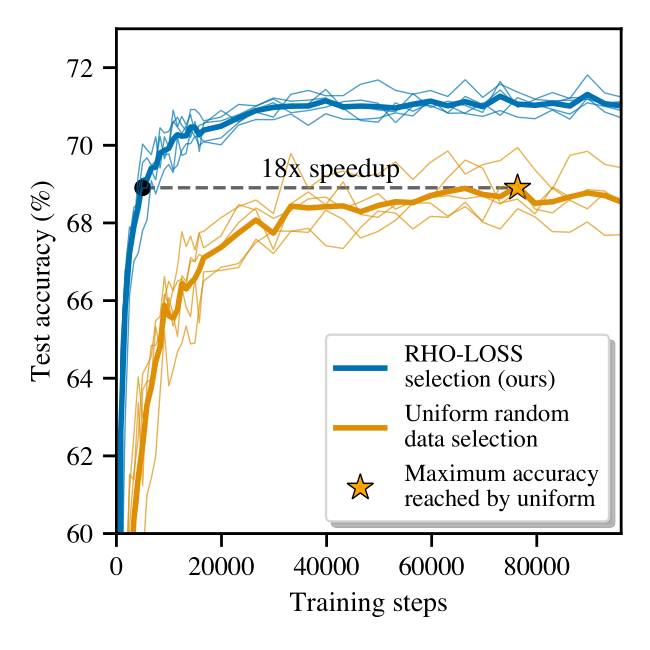
Sören Mindermann, Muhammed Razzak, and Jan Brauner speak at Meta AI Research
21 Sep 2022
OATML PhD Students Sören Mindermann, Muhammed Razzak, and Jan Brauner delivered a talk to Meta AI Research on their work on prioritised training.
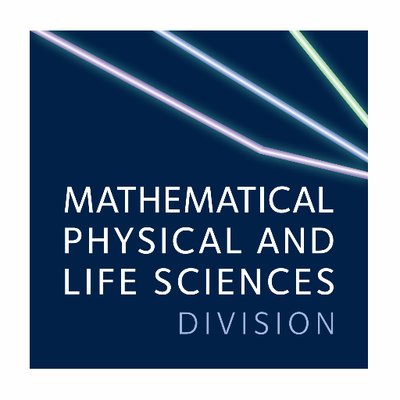
OATML members win Oxford Divisional Impact Award
11 Jul 2022
Prof. Gal, Jan Brauner, Sören Mindermann and Mrinank Sharma have won the MPLS Social Impact Award for their work on evaluating the effectiveness of Covid-19 internentions. Congratulations to all those awarded!
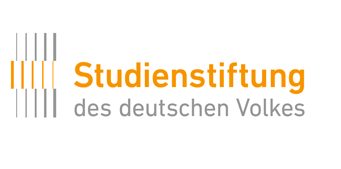
Jan Brauner presents to the German Academic Scholarship Foundation
17 Jun 2022
OATML PhD student Jan Brauner was a guest speaker at an event of the German Academic Scholarship Foundation (10th - 12th June 2022). He gave several talks and workshops on risks from advanced AI systems.
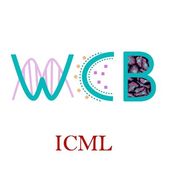
OATML to co-organize the Workshop on Computational Biology at ICML 2022
04 May 2022
OATML student Pascal Notin is co-organizing the 7th edition of the Workshop on Computational Biology (WCB) at ICML 2022 jointly with collaborators at Harvard, Columbia, Cornell and others. OATML students Neil Band, Freddie Bickford Smith, Jan Brauner, Andreas Kirsch and Lood van Niekirk are part of the PC.
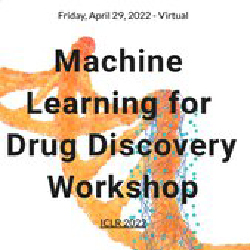
OATML to co-organize the Machine Learning for Drug Discovery (MLDD) workshop at ICLR 2022
15 Jan 2022
OATML students Pascal Notin, Andrew Jesson and Clare Lyle, along with OATML group leader Professor Yarin Gal, are co-organizing the first Machine Learning for Drug Discovery (MLDD) workshop at ICLR 2022 jointly with collaborators at GSK, Harvard, MILA, MIT and others. OATML students Neil Band, Freddie Bickford Smith, Jan Brauner, Lars Holdijk, Andreas Kirsch, Jannik Kossen and Muhammed Razzak are part of the PC.
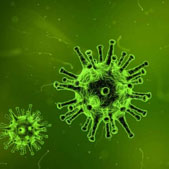
Jan Brauner to speak at the OECD Global Science Forum
24 Sep 2021
Jan Brauner will speak at the OECD Global Science Forum workshop on “Priority setting and coordination of research agendas: lessons learned from COVID 19”. The workshop will take place on October 4th and 5th, further information and a registration link can be found here.
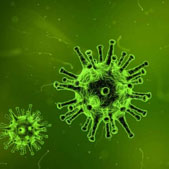
OATML researchers advise UK Cabinet Office
24 Sep 2021
Jan Brauner and Sören Mindermann have presented their work with Professor Yarin Gal and other collaborators on the effectiveness of mask-wearing at reducing COVID-19 transmission to the UK Cabinet Office and advised the office on mask-wearing policies.
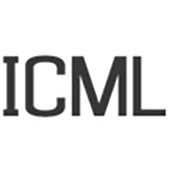
ICML 2021
17 Jul 2021
Seven papers with OATML members accepted to ICML 2021, together with 14 workshop papers. More information in our blog post.
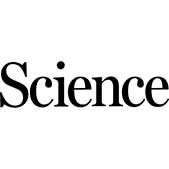
OATML researchers publish paper in Science
15 Dec 2020
The paper called “Inferring the effectiveness of government interventions against COVID-19” was published in Science today. The work is a collaboration with researchers from 9 universities, led by OATML graduate students Sören Mindermann and Jan Brauner, together with Mrinank Sharma from the Department of Statistics.
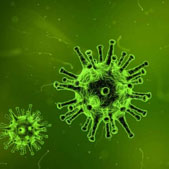
OATML researchers invited to speak at the German Centre for Infection Research
26 Oct 2020
OATML graduate students Sören Mindermann and Jan Brauner, together with Mrinank Sharma from the Department of Statistics, were invited to give a talk about their work with Professor Yarin Gal on ‘inferring the effects of non-pharmaceutical interventions against COVID-19’, at the German Centre for Infection Research/University of Cologne.
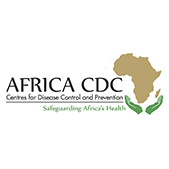
OATML researchers to speak at Africa CDC on COVID-19
06 Jun 2020
OATML graduate students Jan Brauner and Sören Mindermann will give an invited talk at Africa CDC, Africa’s intercontinental public health agency, on June 12. They will present their work with Professor Yarin Gal on nonpharmacetical interventions against COVID-19 to the COVID-19 modelling group.
Blog Posts
OATML at ICML 2022
OATML group members and collaborators are proud to present 11 papers at the ICML 2022 main conference and workshops. Group members are also co-organizing the Workshop on Computational Biology, and the Oxford Wom*n Social. …
Full post...Sören Mindermann, Jan Brauner, Muhammed Razzak, Andreas Kirsch, Aidan Gomez, Sebastian Farquhar, Pascal Notin, Tim G. J. Rudner, Freddie Bickford Smith, Neil Band, Panagiotis Tigas, Andrew Jesson, Lars Holdijk, Joost van Amersfoort, Kelsey Doerksen, Jannik Kossen, Yarin Gal, 17 Jul 2022
21 OATML Conference and Workshop papers at ICML 2021
OATML group members and collaborators are proud to present 21 papers at ICML 2021, including 7 papers at the main conference and 14 papers at various workshops. Group members will also be giving invited talks and participate in panel discussions at the workshops. …
Full post...Angelos Filos, Clare Lyle, Jannik Kossen, Sebastian Farquhar, Tom Rainforth, Andrew Jesson, Sören Mindermann, Tim G. J. Rudner, Oscar Key, Binxin (Robin) Ru, Pascal Notin, Panagiotis Tigas, Andreas Kirsch, Jishnu Mukhoti, Joost van Amersfoort, Lisa Schut, Muhammed Razzak, Aidan Gomez, Jan Brauner, Yarin Gal, 17 Jul 2021
22 OATML Conference and Workshop papers at NeurIPS 2020
OATML group members and collaborators are proud to be presenting 22 papers at NeurIPS 2020. Group members are also co-organising various events around NeurIPS, including workshops, the NeurIPS Meet-Up on Bayesian Deep Learning and socials. …
Full post...Muhammed Razzak, Panagiotis Tigas, Angelos Filos, Atılım Güneş Baydin, Andrew Jesson, Andreas Kirsch, Clare Lyle, Freddie Kalaitzis, Jan Brauner, Jishnu Mukhoti, Lewis Smith, Lisa Schut, Mizu Nishikawa-Toomey, Oscar Key, Binxin (Robin) Ru, Sebastian Farquhar, Sören Mindermann, Tim G. J. Rudner, Yarin Gal, 04 Dec 2020